- The Uncertainty Project
- Posts
- 📘 Artificial Intelligence vs Human Decision Making
📘 Artificial Intelligence vs Human Decision Making
Uniquely human factors, confidence, and the power of team forecasting
In case you missed it, last week we talked about how to better communicate decisions, why we tend to think others share our same beliefs, and how envisioning future failures can help with decision making.
This week:
🤖 Topic: Humans, Artificial Intelligence, and Decision Making
📉 Bias 5/50: Dunning-Kruger Effect
🔮 Tool: Mini-Delphi Method
This was a fun one, so let’s get into it!
Humans, Artificial Intelligence, and Decision Making
Today, aside from rules engines, AI isn’t making many decisions, but what’s the difference between humans and machines when it comes to reasoning and making decisions? How long before machines have the same capabilities - or is it even possible to replicate humans?
According to Hans Moravic, the namesake of the Moravic Paradox, robots will be as smart or surpass human intelligence by 2040, and eventually, as the dominant species, they will merely preserve us as a living museum to honor the species that brought them into existence.
Sounds like Hans wasn’t very fun.
The more optimistic point of view is that human intelligence, paired with the little we know about consciousness, emotion, and the three pounds of mush between our ears, is quite unique.
So while we humans are still calling the shots around here, we’re digging into a few topics around how human decision making differs from a machine.
If biases are ‘bad’, why do we have them?
In this newsletter, we’ve been exploring cognitive biases and techniques to limit their impact on the decision making process - particularly in groups.
Biases are hardwired and as we mentioned in an earlier post, counterarguments suggest the methods used to test their ‘negative’, irrational effects do not take into account many meaningful, real-world factors.
We make strategic decisions in environments of extreme uncertainty with fierce competition. There are many confounding variables outside of our control that we generally label as ‘luck’.
This point of view starts to surface plenty of interesting questions…
Why are emotion, trust, competition, and perception meaningful factors in making decisions?
Why do we hold irrational convictions and have trouble thinking probabilistically?
Why are we optimized for this ability to model our environment off of very little information?
Why does ‘investigative’, abductive reasoning come so naturally to us?
Gary Klein, Gerd Gigerenzer, Phil Rosenzweig, and others make the argument that these things that make us very human, hold the secret to how we make complex, highly consequential decisions in high-speed, low-information situations.
To be clear, there’s a strong overlap where both camps agree. In a 2010 interview, Kahneman and Klein debated the two points of view:
Both agree that explicit decision making processes are important, particularly when evaluating information.
Both believe intuition can and should be used, though Kahneman stresses it should be delayed as long as possible.
Both agree that domain expertise matters, but Kahneman argues biases are particularly strong in experts and must be corrected.

So why do our brains rely so heavily on biases and heuristics?
Our brains optimize for energy consumption. They consume roughly 20% of the energy we produce in a day - and to think Aristotle thought the brain’s primary function was simply a radiator to keep the heart from overheating.
Silly Aristotle.
From there, energy consumption within the brain is a black box, but research suggests, in general, the functions that require a lot of processing, such as complex problem-solving, decision making, and working memory, tend to use more energy than functions that are more routine or automatic, like breathing and digesting.
For this reason, the brain tends to not make decisions. It does this by creating structures for what Daniel Kahneman calls ‘system 1’ thinking. These structures use cognitive ‘shortcuts’ (heuristics) to make energy-efficient decisions that feel conscious but rely on a foundation of subconscious functions. When we elevate decisions that need more cognitive power, Kahneman calls this ‘system 2’ thinking.
Since Kahneman’s book Thinking, Fast and Slow is an incredibly popular New York Times best-seller, this may be a review, but this is what we’re typically taught: Biases and heuristics impair decision making - that intuition is often flawed in human judgment.
There’s a counterargument to the biases and heuristics model proposed by Kahneman and Amos Tversky, and it’s based on the fact that their studies were done in controlled, lab-like environments with decisions that have relatively certain outcomes (as opposed to the often complex, consequential decisions we make in life and work)
These arguments broadly fall under Ecological-Rationality and Naturalistic Decision Making (NDM). In short, they generally argue the same thing: Humans, armed with these heuristics, often rely on recognition-primed decision making. The recognition of patterns in our experiences helps us make decisions quickly and effectively in these high-stakes, highly uncertain situations.
Humans are quite good at extrapolating very little information into models for decision making based on our experiences - regardless of whether or not the judgments we make, on their own, are objectively correct, we have this ability to strategize.
As the founder of Deepmind, Demis Hassabis, expressed in an interview with Lex Friedman, as these intelligent systems become more intelligent, it makes it easier to understand what makes human cognition different.
There seems to be something deeply human about our ability to ask ‘why’, perceive meaning, have the conviction to act, and maybe most importantly - do this in groups.
“Human intelligence is largely externalized, contained not in your brain but in your civilization. Think of individuals as tools, whose brains are modules in a cognitive system much larger than themselves—a system that is self-improving and has been for a long time.” - Erik J. Larson, The Myth of Artificial Intelligence: Why Computers Can’t Think the Way We Do
Though the last 50 years have rendered incredible leaps in understanding how we make decisions, it may be artificial intelligence, through its limitations, that uncovers more about the power of human cognition.
Or humanity becomes the Tamagotchis of our robot overlords…
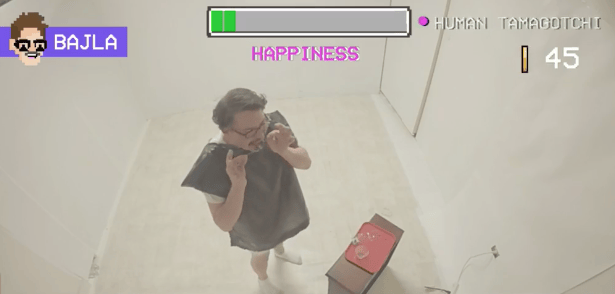
Bias 5/50: the Dunning-Kruger Effect
The Dunning-Kruger effect refers to the phenomenon of people who are relatively incompetent in a domain having an inflated sense of their own ability or knowledge. Conversely, those who have high competence in a domain often have a lower sense of their own ability to knowledge.
Researchers believe this is because the less we know, the more we think we know - and the more we know, the more we realize we don’t know.
The more you know, the more you realize you don't know.
Put some points back on the board for Aristotle!
Within teams and organizations, there’s rarely, if ever, any explicit measure of merit. Not to say this is a bad thing - it doesn’t feel natural or feasible to have some kind of ‘score’ that illustrates how reliable someone’s point of view is in a specific domain - but this means we often rely on the signal of confidence.
When someone exudes confidence, we believe them.
The Dunning-Kruger effect illustrates why this is a problem, and not only from the more obvious perspective of false confidence but those with the highest competency are often less confident.
This is where tools like the mini-Delphi method or nominal group collaboration can help tease out where overconfidence might be eclipsing better-informed, but more skeptical points of view.
Is over-confidence ever useful?
Daniel Kahneman’s harps on this concern of overconfidence in strategic decision making - particularly across domains.
Phil Rosenzweig, in his book ‘Left Brain, Right Stuff’, argues that studies on decision making fail to capture the effect of competition and conviction. He uses the example of high-performing athletes to argue that over-confidence “isn’t just useful, it’s essential from a competitive perspective”, and business is a competitive environment.
If someone has a level of confidence that slightly exceeds that which is objectively warranted, that isn’t a bad thing. That’s how you will improve your performance.
Of course, the two agree on mitigating extreme hubris that can lead to rash decision making - and recognize the posthoc resulting problem of attributing ‘overconfidence’ when it fails and ‘genius’ when it succeeds - but the nuance is interesting.
Confidence may be a tool in this highly competitive, uncertain environment as long as it’s kept in check - particularly when working in groups.
The Mini-Delphi Method
How might we ‘query’ the wealth of knowledge in teams or organizations and avoid the confidence problem? This method is an interesting way to make the process explicit.
The mini-Delphi method is a variant of the Delphi method, a group decision making technique that is used to gather and integrate the opinions of a group of ‘experts’ - typically in the form of probabilistic forecasts paired with levels of confidence.
In this case, back to our point about merit, ‘expert’ is used loosely.
It’s essentially a simplified version of the Delphi method that can be used with a smaller number of experts and with less time and resources.
How to use the mini-Delphi method
The mini-Delphi method, also referred to as ‘estimate-talk-estimate’, is a particular form of nominal group collaboration typically used to survey panels of experts, so it’s no surprise the steps are similar between the two methods.
Unlike general nominal group collaboration, the mini-Delphi method is typically used to formulate a collective probabilistic forecast from a group of experts.
To summarize the process, a small group of experts is asked to provide their opinions on a specific issue or question through a series of rounds. Each round begins with the experts providing their initial opinions, which are then anonymously compiled and shared with the group. The experts then have the opportunity to review and revise their opinions in light of the group's input. This process is repeated for a predetermined number of rounds, typically two or three.
The typical process for the mini-Delphi looks like this:
Define the problem or question to be addressed by the group: As with a typical mini-Delphi, if we’re seeking a ‘forecast’ from experts, framing the question is an important, non-trivial step.
Select a group of experts or stakeholders who have relevant knowledge or experience: The more this process can be automated through surveys, the more opinions/forecasts can be collected. For the ‘wisdom of crowds’ approach, this can be expanded accordingly.
Ask each member of the group to provide anonymous written responses: This would typically include the forecast, the proposed probability of the forecast, and the supporting argument/information.
Collect and compile the responses into a summary report: At the very least, this is a readout that summarizes the responses but may also include the average of the probabilistic forecasts or groupings of similar responses.
Share the summary report with all members of the group and ask them to provide feedback on the responses: Participants should have the ability to comment on the individual responses of others as well as the summary of the report. Participants can revise their own responses async or in real-time.
Collect and re-compile the feedback into a final report, which should include a summary of the main findings and recommendations: This process can be repeated (often 2-3 times) if needed before compiling a final report
Again, the mini-Delphi method is typically used for collecting and aggregating forecasts from groups of experts or to leverage the wisdom of crowds. For standard collaboration where we may just be requesting information, surfacing dissent, or getting feedback from groups, using nominal groups would suffice.
Bonus: saving and building on these forecasts
At this point, we might have a series of forecasts by individuals. Instead of just running a mini-Delphi over and over, we can retain these forecasts and start to build a library that can be referenced or updated by others.
The team can continue to uncover new information and update their beliefs in real time as these forecasts continue to shape decisions in the future.
Forecast, measure, revise: it is the surest path to seeing better.
How was this week's post?We'd love to know what you think! (click one) |
Reply